DOWNLOAD PDF
October 18, 2019
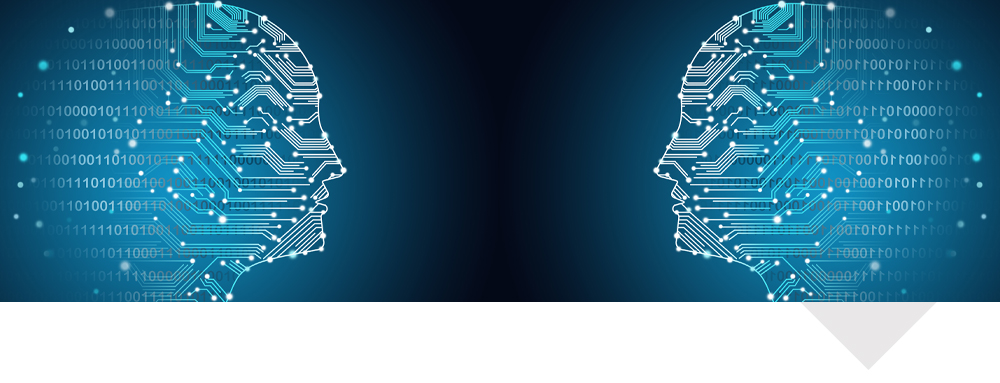
IntroductionArtificial intelligence (AI) is an intriguing emerging technology. Rapidly adopted by the IT industry, it has found myriad applications in our economy and now appears to be ubiquitous. Its capacity to automate processes and extend the reach of the human mind is already causing profound changes in a number of fields, including auditing. This article explores how AI is revolutionizing the audit profession by expanding the range, efficiency, and extent of audit evidence collection and analysis. It describes the main applications of AI to auditing and discusses the opportunities and challenges that AI presents for public sector auditors. What is AI?Artificial intelligence is surrounded by a fair amount of hype. Like all technological trends, it generates considerable enthusiasm and skepticism. Yet AI is far from a passing science-fiction fad and has solid academic roots. The term “AI” was coined in 1955 by a professor of computer science at Dartmouth College, John McCarthy, to describe what was already a vibrant and ambitious multidisciplinary field of research. In his words, the study of AI proceeded “on the basis of the conjecture that every aspect of learning or any other feature of intelligence can in principle be so precisely described that a machine can be made to simulate it.”1 He further speculated that machines would use language, form abstractions and concepts, and solve problems reserved for humans. These prophetic musings sound eerily true to 21st-century readers. AI’s fate and success have waxed and waned through a number of cycles since the 1950s. AI has, through these ups and downs, been used in a number of ways, from appliances to Internet search engines. In the last few years it seems to be undergoing a breathtaking explosion. This “AI Revolution” has been fuelled by quantum leaps in:
Every day, announcements are made about the use of AI in new areas. For example:
A lot of these AI-driven innovations are now familiar to many consumers and are becoming run-of-the-mill technologies in various industries. But a number of them are still in their infancy and very far from being proven, mainstream technologies. As seen in Figure 1, AI capabilities have diversified in recent years and can now be applied to various tasks. This diversification has been made possible by exploiting:
Use of AI in AuditingThe audit profession has started to find many ways to integrate AI in its operations. Some authors have provided exhaustive inventories of potential uses of AI by auditors.2 In this article, we will focus on three areas that are fuelling the most promising technologies for auditors: image recognition, speech recognition, and machine learning. Image RecognitionImage recognition is possible because AI can process video and digital images mathematically and identify patterns emerging from the data. Bar code scanning and Apple’s face recognition technology on laptops and devices are well-known applications of this technology. In its more advanced form, image recognition can automate routine audit procedures. For example, it can do inventory counts using a video camera attached to drones, identify and locate items, and even report on their condition. Such video analytics can be performed very efficiently, reviewing hours of recordings in a few minutes. Performance auditors can also use this technology. For example, satellite imagery has been used by the European Court of Auditors to facilitate checks on, for instance, crop classification and the agricultural activity taking place on land parcels.3 This capacity to process images is changing the way auditors implement audit procedures, such as observations and inspections.4 A detailed overview of this technology, together with a case study of its implementation, can be found here. Speech RecognitionSpeech recognition, another subfield of AI, aims to leverage streamed or recorded audio such as voice mails, phone calls, conference calls, interviews, management presentations, and recording of management or board of directors’ meetings. Speech recognition software “understands” and analyzes grammatical and semantical language structures, taking into account accents, errors, and background noise. Speech recognition applications are widely used in mobile telephony, video games, and home automation, to mention a few. A promising audit application is the ability to accurately transcribe verbal recordings of interviews and testimonies, thus efficiently generating reliable transcripts of conference calls or interviews.5 More importantly, this technology can understand and analyze the language used by the interviewees, speakers, or participants, to infer emotional states, attempts at deception, or uncertainty of answers.6 A good overview of the literature on this topic is available here. Machine LearningMachine learning is an advanced form of AI. It is the engine that can leverage the other subsets of AI mentioned above and it conforms most closely with the popular concept of a machine replacing humans. Machine learning uses algorithmic models to perform complex data analysis on large datasets without specific instructions. It is programmed to iteratively process the data and build mathematical models, in order to identify patterns and make predictions.7 In its most advanced version, known as deep learning, AI is operated by neural networks emulating the human brain and can help make decisions and diagnostics.8 Applications using this technology are emerging in the audit profession. For example, software that can read complex contracts,9 identify key terms, and correlate with transactions could identify patterns and instances of non-compliance. An example of a commercially available audit solution is AI Audit by MindBridge,10 which assesses risks of fraud, identifies data anomalies, and examines full transactions, looking at the monetary flows between accounts. Also, software like the Auditors Deep Learning Dashboard by SageTea11 and AuditMap by Lemay.ai12 aim to analyze risks and controls, and generally help the planning and conduct of audits. Opportunities and Challenges for AuditorsAuditing AI TechnologiesBefore AI becomes a staple of the audit toolbox, auditors will be made aware of it through its presence in multiple operations of the organizations they audit. This will demand that audits take AI into account, or even provide assurance on its functioning. Therefore, as organizations deploy AI, auditors will be asked to assess the risks and vulnerabilities of the technologies themselves.13 Auditing AI technologies presents an array of challenges for auditors.
However, efforts to provide audit frameworks are starting to emerge.15 Skills Needed by Auditors to Succeed in an AI WorldTo audit and use AI, auditors will need the technical knowledge and tools to assess this technology and its impact on the economy, efficiency, and effectiveness of auditees. Therefore, auditors will have to hone and perfect skills they already have while acquiring new ones.16 The most relevant skills needed are:
AI BiasesObjectivity is paramount for auditors. It is the very foundation of auditing standards and of auditors’ credibility. Therefore, concerns about AI biases are both a risk to auditees and a constraint on the use of AI in audits. At the end of the day, AI is based on code and is bound to reflect and even amplify the biases of coders. There are many examples of AI biases, such as software powered by AI used in Florida to assess inmates’ risks of recidivism.17 It was found that with this software, black defendants were far more likely than white defendants to be incorrectly judged to be at a higher risk of recidivism. Another example is an algorithm used to score New York City landlords on how well they take care of their properties, which was shown by an audit to be adversely impacted by biases in the inspection reports on which it relied.18 These biases are magnified by the limitations of the neural network technology underpinning deep learning. Some AI programs produce decisions and actions that are fundamentally unexplained and unexplainable. For example, a research group at Mount Sinai Hospital in New York created a deep learning application named Deep Patient to analyze a vast database of 700,000 individuals. It proved very effective at predicting health issues. For instance, it was remarkably effective at anticipating the onset of schizophrenia. However, when the researchers tried to identify how Deep Patient was piecing data together to reach its conclusions, they could not. This made it impossible to understand the rationale of the diagnostic and therefore the justification for a specific treatment or a change in medication.19 Interestingly, this phenomenon, frequently called the “black box” effect, has been recognized by the audit profession.20 Efforts are ongoing to try to identify, trace, and document the decision-making process of AI using deep learning. The ability to document the logic behind a conclusion reached by an AI application would be of great value to performance auditors, who must support their conclusions with sufficient and appropriate evidence. How AI Can Enhance the Auditor’s RoleAI shines especially well when undertaking tedious tasks. The audit profession entails a number of “low-hanging fruits” in this respect: contract reviews, inventories, compliance reviews of transactions, and so on. AI can do all that better than humans. It is faster, efficient, and accurate, and can often review 100% of transactions.21 Some studies predicted that up to 40% of transaction accounting work would be automated or eliminated by 2020.22 Humans can also be surpassed by AI’s ability to find patterns that would be undetectable otherwise, which is particularly suited to detecting fraud or conducting root-cause analysis. The effort to develop self-driving cars has also led to the development of ethical algorithms that can make decisions based on an ethical framework.23 This creates the possibility of reviewing minutes of meetings or reports and other corporate documents to identify ethical breaches. Although the automation of tasks requiring professional judgment is still imperfect, the automation of tedious tasks is underway. This can enhance and extend the role of humans, not eliminate it.24 Humans are still better than computers at telling the story behind the data and at making qualitative judgments that add value for auditees. ConclusionThe roots of artificial intelligence in computer science go too far and its modern applications are too important for it to be a passing fad. It is already fundamentally changing our society and our economy. Despite its challenges, it will fundamentally change the way audits are conducted. This article barely scratched the surface of this complex technology but hopefully provided useful suggestions of where to dig for more knowledge of AI. Like in many other fields, AI will not replace professionals, but if properly harnessed, it will enhance their capacity to deliver quality products and services. |
Author
Yves Genest, Vice-President, Products and Services, CAAF. You can send your questions and comments on this article to the author at research@caaf-fcar.ca.
References
Axson, D. A. J. (2015). Finance 2020: Death by Digital. Accenture, available at: https://www.accenture.com/t20150902t015110__w__/us-en/_acnmedia/accenture/conversion-assets/dotcom/documents/global/pdf/dualpub_21/accenture-finance-2020-pov.pdf
Chartered Professional Accountants of Canada (CPA Canada) (2019a). “A CPA’s Introduction to AI: From Algorithms to Deep Learning,” available at: https://www.cpacanada.ca/en/business-and-accounting-resources/other-general-business-topics/information-management-and-technology/publications/a-cpa-introduction-to-ai
Chartered Professional Accountants of Canada (CPA Canada) (2019b). “How Are Big Data and AI Transforming Accounting and Finance?,” available at: https://www.cpacanada.ca/en/business-and-accounting-resources/other-general-business-topics/information-management-and-technology/publications/ai-impact-on-accounting-and-finance
Christ, M. H., et al. (2019, March 7). “Prepare for Takeoff: Improving Asset Measurement and Audit Quality with Drone-Enabled Inventory Audit Procedures.” SSRN, available at: https://ssrn.com/abstract=3335204
Davenport, T. (2016, February 24). “Innovation in Audit Takes the Analytics, AI Route: Audit Analytics, Cognitive Technologies to Set Accountants Free from Grunt Work.” Deloitte Insights, available at: https://www2.deloitte.com/insights/us/en/focus/cognitive-technologies/audit-analytics-artificial-intelligence.html
Davenport, T. and J. Raphael (2017, July 12). “Creating a Cognitive Audit.” CFO, available at: https://www.cfo.com/auditing/2017/07/creating-cognitive-audit/
Drew J. (2019, June 1). “What’s ‘Critical’ for CPAs to Learn in an AI-Powered World.” Journal of Accountancy, available at: https://www.journalofaccountancy.com/issues/2019/jun/critical-cpa-skills-for-ai-powered-world.html
Etzioni, O. and M. Li (2019, July 18). “High-Stakes AI Decisions Need to Be Automatically Audited.” Wired, available at: https://www.wired.com/story/ai-needs-to-be-audited/
Fisher, I., M. Garnsey, and M. Hughes (2016). “Natural Language Processing in Accounting, Auditing and Finance: A Synthesis of the Literature with a Roadmap for Future Research.” Intelligent Systems in Accounting, Finance and Management, volume 23, number 3, available at: https://www.researchgate.net/publication/296469200_Natural_Language_Processing_in_Accounting_Auditing_and_
Finance_A_Synthesis_of_the_Literature_with_a_Roadmap_for_Future_Research
Guszcza, J., et al. (2018, November 28). “Why We Need to Audit Algorithms,” Harvard Business Review, available at: https://hbr.org/2018/11/why-we-need-to-audit-algorithms
Hempel, J. (2018, May 9). “Want to Prove Your Business Is Fair? Audit Your Algorithm.” Wired, available at: https://www.wired.com/story/want-to-prove-your-business-is-fair-audit-your-algorithm/
Institute of Internal Auditors (IIA) (2017). Global Perspectives and Insights, Artificial Intelligence: Considerations for the Profession of Internal Auditing, Special Edition, available at: https://na.theiia.org/periodicals/Public%20Documents/GPI-Artificial-Intelligence.pdf
Institute of Internal Auditors (IIA) (2018). Global Perspectives and Insights, The IIA’s Artificial Intelligence Auditing Framework Practical Applications: Part B, Special Edition, available at: https://na.theiia.org/periodicals/Public%20Documents/GPI-Artificial-Intelligence-Part-III.pdf
ISACA (2018). Auditing Artificial Intelligence, available at http://www.isaca.org/Knowledge-Center/Research/ResearchDeliverables/Pages/Auditing-Artificial-Intelligence.aspx
Issa, H., T. Sun, and M. A. Vasarhelyi (2016). “Research Ideas for Artificial Intelligence in Auditing: The Formalization of Audit and Workforce Supplementation.” Journal of Emerging Technologies in Accounting, volume 13, number 2, available at: https://aaajournals.org/doi/pdf/10.2308/jeta-10511
Knight, W. (2017, April 11). “The Dark Secret at the Heart of AI.” MIT Technology Review, available at: https://www.technologyreview.com/s/604087/the-dark-secret-at-the-heart-of-ai/
Kundu S. (2019, July 3). “Ethics in the Age of Artificial Intelligence: If We Don’t Know How AIs Make Decisions, How Can We Trust What They Decide?” Scientific American, available at: https://blogs.scientificamerican.com/observations/ethics-in-the-age-of-artificial-intelligence/
Larson, J., S. Mattu, L. Kirchner, and J. Angwin (2016, May 23). “How We Analyzed the COMPAS Recidivism Algorithm.” Pro Publica, available at: https://www.propublica.org/article/how-we-analyzed-the-compas-recidivism-algorithm
Maxmen, A. (2018, October 24). “Self-Driving Car Dilemmas Reveal that Moral Choices Are Not Universal.” Nature – International Journal of Science, available at: https://www.nature.com/articles/d41586-018-07135-0
Mayew, W. and M. Venkatachalam (2013). “Speech Analysis in Financial Markets.” Foundations and Trends in Accounting, volume 7, number 2, pp. 73–130. Abstract available at: https://www.nowpublishers.com/article/Details/ACC-024
McCarthy, J., et al. (2006). “A Proposal for the Darmouth Summer Research Project on Artificial Intelligence, August 31, 1955.” AI Magazine, volume 27, number 4, available at: https://aaai.org/ojs/index.php/aimagazine/article/view/1904/1802
McKendrick, J. (2019, June 28). “Artificial Intelligence May Amplify Bias, But Also Can Help Eliminate It.” Forbes, available at: https://www.forbes.com/sites/joemckendrick/2019/06/28/artificial-intelligence-may-be-biased-but-it-can-also-help-bust-bias/#2dcec79f22d0
McKinsey Global Institute (2017, June). Artificial Intelligence: The Next Digital Frontier?, available at: https://www.mckinsey.com/~/media/McKinsey/Industries/Advanced%20Electronics/
Our%20Insights/How%20artificial%20intelligence%20can%20deliver%20real%20value%20to%20companies/MGI-Artificial-Intelligence-Discussion-paper.ashx
Patriquin, M. (2019, February 26). “When the Machines Take Over.” Pivot Magazine, available at: https://www.cpacanada.ca/en/news/pivot-magazine/2019-02-26-mindbridge-ai-auditing
Persico, F. (2017, April 5). “How AI Will Turn Auditors into Analysts.” Accounting Today, available at: https://www.accountingtoday.com/opinion/how-ai-will-turn-auditors-into-analysts
PwC (2018). 2018 AI Predictions: 8 Insights to Shape Business Strategy, available at: https://www.pwc.es/es/home/assets/ai-predictions-2018-report.pdf
Raphael, J. (2015, June 15). “How Artificial Intelligence Can Boost Audit Quality.” CFO, available at: https://www.cfo.com/auditing/2015/06/artificial-intelligence-can-boost-audit-quality/
Romanini, F. (2018, March 15). “Is Artificial Intelligence and Machine Learning the Future of Financial Regulation?” LinkedIn, available at: https://www.linkedin.com/pulse/artificial-intelligence-machine-learning-future-franco-romanini-/
Sun, T. and M. A. Vasarhelyi (2017, June). “Deep Learning and the Future of Auditing: How an Evolving Technology Could Transform Analysis and Improve Judgment.” The CPA Journal, available at: https://www.cpajournal.com/2017/06/19/deep-learning-future-auditing/
Thompson, P. (2017, June 28). “Digital Technologies’ Implications for SMPs.” International Federation of Accountants, available at: https://www.ifac.org/global-knowledge-gateway/practice-management/discussion/digital-technologies-implications-smps
Torres, M. (2018, May 18). “This New AI Service Will Transcribe Conference Calls in Real-Time.” Ladders.com, available at: https://www.theladders.com/career-advice/this-new-ai-service-will-transcribe-conference-calls-in-real-time-for-you
Endnotes
1 McCarthy, J., et al. (2006).
2 Issa, H., T. Sun, and M. A. Vasarhelyi (2016).
3 European Court of Auditors (2019), EU Auditors look at new imaging technologies for monitoring the CAP, https://www.eca.europa.eu/en/Pages/NewsItem.aspx?nid=12475
4 Sun, T. and M. A. Vasarhelyi (2017).
5 Torres, M. (2018).
6 Mayew, W. and M. Venkatachalam (2013).
7 CPA Canada (2019a).
8 Sun, T. and M. A. Vasarhelyi (2017).
9 Patriquin, M. (2019).
10 “AI Auditor,” MindBridge website, https://www.mindbridge.ai/products/ai-auditor/.
11 “Auditors Deep Learning Dashboard,” SageTea website, https://sagetea.ai/product/auditors-deep-learning-dashboard/.
12 AuditMap website, https://auditmap.ai/.
13 Guszcza, J., et al. (2018).
14 ISACA (2018).
15 IIA (2018).
16 Drew, J. (2019).
17 Larson, J., S. Mattu, L. Kirchner, and J. Angwin (2016).
18 Hempel, J. (2018).
19 Knight, W. (2017).
20 IIA (2018).
21 Davenport, T. (2016).
22 Axson, D. A. J. (2015).
23 Maxmen, A. (2018).
24 Persico, F. (2017).
See more Research Highlights