April 14, 2023
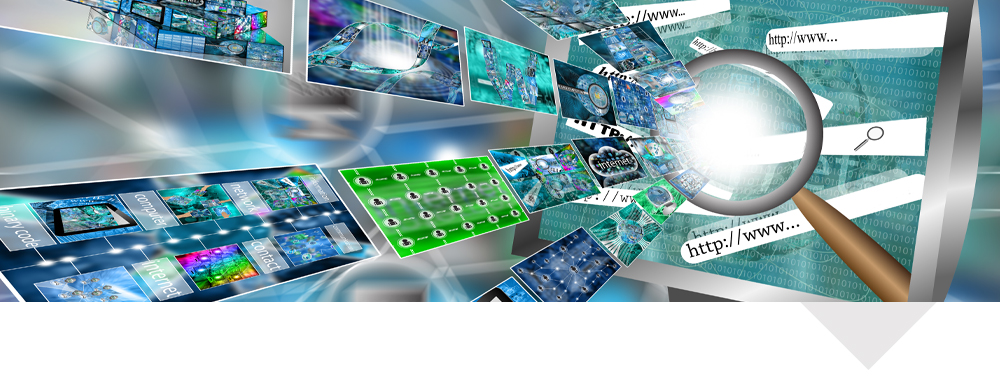
The digital age has created an abundance of raw data, which if understood, would be a useful asset to decision-makers at organizations. Yet, while there has been great progress in gathering business intelligence from structured data, analyzing unstructured data such as written information is still challenging. For example, unstructured data comprises most of the data within organizations, and manually processing such high volumes of data can be labor-intensive and time-consuming. Examples of unstructured textual data include interview notes, web articles, and text within any document or email.
The Canada Revenue Agency (CRA) is using a form of artificial intelligence — natural language processing (NLP) — to analyze this unstructured, textual data. The objective of NLP is to read, decipher, understand, and make sense of human language in a manner that is valuable. The technology has enabled internal auditors at the CRA to understand and analyze high volumes of unstructured textual data contained in interview notes, web articles, and final reports. The CRA has found NLP particularly effective for summarizing, modeling topics, and analyzing sentiment.
Automatic Text Summarization
In 20 minutes, auditors were able to process notes from about 100 interviews containing approximately 140,000 words. |
Summarizing text within documents is time-consuming. Using NLP to automate that process reduces large documents to a fraction of their original size while retaining most of their meaning. Moreover, automatic text summarization can increase the speed at which internal auditors review documents while reducing the effort and time needed to manually read and prepare summaries.
The agency automatically scans web articles based on search terms and applies automatic text summarization to produce relatively accurate summaries. In most cases, large documents are reduced to less than one-tenth of their original size, making it faster for practitioners to complete their initial reviews. The CRA's Enterprise Risk Management Division, for example, uses these summaries to help report risk information, and so far, the reaction has been positive.
Additionally, the CRA has explored two opportunities to use automated text summaries in the planning phase of an engagement. One application is processing and summarizing a large volume of interview notes for an organization wide review. In roughly 20 minutes, auditors were able to process notes from about 100 interviews containing approximately 140,000 words. The second application is summarizing a large body of internal corporate information stored on the CRA's intranet to accelerate the gathering of existing internal controls such as mandates, policies, and procedures related to the specific audit entity.
Topic Modeling and Topic Visualization
Topic modeling is the process of classifying the important topics and themes contained in a body of text or a collection of documents. Using NLP for topic modeling can provide an analyst a wide range of overall themes or topics at a speed and scope of detail that surpasses that of a person. That can save analyst the time and effort of manually classifying documents according to their logical categories.
Additionally, auditors can visualize topics within documents to reveal their prevalence as well as the correlation strengths between topics and documents. For example, risk analysts at the CRA used topic visualization methods to automatically uncover topics within risk statements and how they are correlated (see "Visualizing Connections," below).
Topic modeling also can be combined with automatic text summaries to help analysts more quickly understand the content within documents and home in on certain themes or topics of interest. For example, a risk analyst might be interested to know how a few dozen online journals and newspapers have reported on the organization's operations over several years. A data analyst could gather news articles that mention the organization and apply NLP algorithms to create a topic model to identify themes of interest. Then the articles could be automatically summarized, enabling the risk analyst to review them rapidly to identify risks.
Visualizing ConnectionsCRA practitioners used the visualization depicted to show the interconnectivity of risk statements to topics (nodes), and the associated correlations strengths (line thickness). In this case, there were 31 risk statements (small nodes) connected to 8 different topics (large nodes). Risk 7 and Risk 14 are strongly associated with Topic 7, but Risk 5 and Risk 1 are less associated with that topic. Risk 1 is more strongly associated with Topic 1. This network diagram shows how risk statements can be categorized into themes (topics), and how certain risk statements are linked to multiple themes.
|
Sentiment Analysis
Sentiment analysis is the process of analyzing written text to determine tone. The tone can be positive, negative, or neutral. NLP automates this process by scanning entire articles at the sentence level to determine the overall tone. For example, auditors can assign words such as great, happy, and friendly to a positive sentiment, and assign words such as terrible, angry, and annoying to a negative sentiment.
Data analysts at the CRA used the R programming language to uncover sentiment from responses to 20 interview questions, which were asked in many interviews. The analysis determined that 15 interview questions elicited a negative response, but only five questions elicited a positive response. One explanation for this is that the questions were worded in a way to uncover weaknesses or problem areas, so a more negative response would be expected. This is an example of why auditors should be careful when using sentiment analysis because the context must be known in advance. Although sentiment analysis can provide a general glimpse about how respondents may feel about a topic, auditors should look for what might explain the sentiment.
The CRA also used sentiment analysis to determine the tone of findings in the agency's publicly available internal audit reports. In cases where balanced reporting was preferred, auditors used sentiment analysis to determine whether reports had a balance of statements showing that some internal controls were functioning well and some needed improvement. By charting scatter plots of findings statements by year, auditors could see the tonal trend in sentiment over time.
Uncovering Knowledge
As the CRA has discovered, NLP can augment the intelligence-gathering efforts of internal auditors by helping them uncover knowledge and connect information across large volumes of textual data. The CRA's Audit, Evaluation, and Risk branch encourages practitioners to use NLP and other AI methods to process and analyze the large volumes of unstructured textual data stored within organizations.
Getting started can be as easy as hiring a student or new graduate with some expertise in leveraging freely available programing tools, such as R and Python. These open source tools and the available AI algorithms are open to scrutiny and continuous improvement. Before using them, internal audit functions should consult with their IT department to ensure that the tools have been configured and certified for internal use by their organizations.
Mourad Nizar, CISA, is director, Professional Practices and Data Analysis Division at the Canada Revenue Agency in Ottawa
Jasbir Singh, CISA, is project leader, Data Analysis Innovation, at the Canada Revenue Agency in Ottawa
jasbir.singh@cra-arc.gc.ca
Francis Hamel is director, Data Services and Analysis, at the Public Service Commission of Canada in Ottawa
DISCLAIMER: The opinions expressed in this article are those of the authors and do not necessarily reflect the views of the Foundation.
This article was reprinted with permission from the April 2022 issue of Internal Auditor, published by The Institute of Internal Auditors, Inc., www.theiia.org.
See more Voices from the Field